AI and the Lab of the Future
Infographic
Published: October 30, 2023
|
Ruairi J Mackenzie
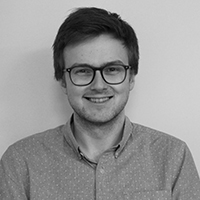
As senior science writer, Ruairi pens and edits scientific news, articles and features, with a focus on the complexities and curiosities of the brain and emerging informatics technologies. Ruairi also drives Technology Networks' search engine optimization (SEO) and editorial AI strategy and created the site’s podcast, Opinionated Science, in 2020. Ruairi has a Master’s degree in Clinical Neurosciences from the University of Cambridge.
Learn about our editorial policies
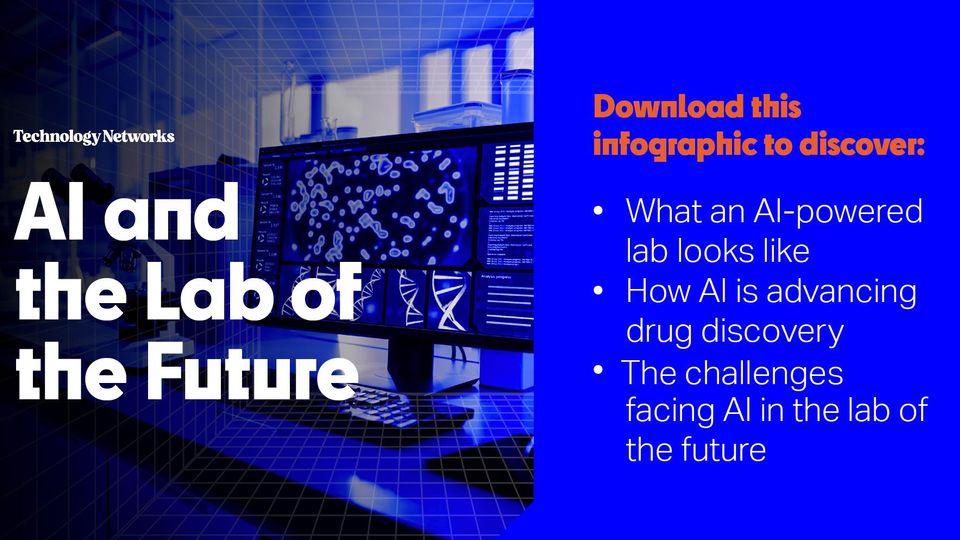
Credit: Technology Networks
In 2023, AI technologies broke into the public consciousness. But for lab leaders, AI tools have been growing in importance over the last decade. In the lab of the future, AI is likely to take an even more significant role in day-to-day processes. In this infographic, we explore some of the potential AI holds for the future of laboratory research.
Download this infographic to discover:
- What an AI-powered lab looks like
- How AI is advancing drug discovery
- The challenges facing AI in the lab of the future
AI and
the Lab of
the Future
In 2023, AI technologies broke into the public consciousness. But for lab leaders, AI
tools have been growing in importance over the last decade. In the lab of the future,
AI is likely to take an even more significant role in day-to-day processes.
A September 2023 survey by the nonprofit Pistoia Alliance
+
looked at the lab technologies that life science companies
intended to invest in over the next two years
c
What is AI?
AI and machine learning (ML) came out on top, ranking as one
AI has become a red-hot
of the top three investment priorities for 60% of companies.
buzzword across science. At its
core, AI is a general term that
covers a range of more specifi
60%
techniques that leverage
computing power to solve data
problems. Let’s take a closer
51%
look:
Machine Learning (ML)
36%
Uses algorithms that are
“trained” to recognize patterns
29%
and themes in datasets. This
enables the algorithms to make
inferences and predictions
about future data they are
presented with
t
Deep Learning
A subset of ML that feeds data
through structured, layered
LIMS
Cloud Data
AI/ML
Robotics/
networks called convolutional
Plataforms
Automation
neural networks (CNNs). This
facilitates more accurate
Many other highly ranked technologies in the survey,
analysis of complex systems.
4
such as robotics and cloud computing platforms, will
come to incorporate AI over the next few years.
Natural Language
Processing (NLP)
A digital approach to studying
text that aims to automatically
process linguistic elements in
the text
Machine Vision
While NLP uses AI techniques
to analyze text, machine vision
studies imaging data. This
involves image processing
techniques like filtering
pixel counting and pattern
recognition
The market is responding to these changing priorities – a
n
recent McKinsey research report identified almost 270
companies working on AI technologies in drug discovery
.2
What Does an AI-Powered Lab Look Like?
AI tools will be at the heart of the lab of the future. The Lawrence Berkeley National Laboratory’s A-Lab is a
powerful demonstration of how AI could be used. A-Lab is a materials lab, carrying out assays on samples
that could be useful in engineering applications such as solar and fuel cell development.
s
+
The robot arms at A-Lab
The robot picks from
can together process 50–
nearly 200 potential
100 times as many samples
precursor molecules.
as a human.
A-Lab
by the
numbers:
Working with
a database of
150,000 new
materials
Robotic arms: 3
Furnaces: 8
Powder
precursors:
~200
Lab size: 600
square feet
Operates: 24/7
Samples tested
per day:
Data from the lab is
100–200
The lab’s central decision
automatically sent to data
making
hub is controlled by
repositories such as the
AI software.
Materials Project
h
AI in Drug Discovery
The field of drug discovery has readily embraced AI. Tools powered by this software can help narrow down
the millions of potential targets for small-molecule and biologic drugs.
Target Screening
+
Assessing physiological targets
for potential drugs involves
AI Tools in Drug
incorporating huge sources of omics
data; AI can help this process
Discovery12
f omics
data; AI can help this process.9
AlphaFold
3D Protein structure prediction
Drug Screening
DeepChem
A python-based library for
ML tools like AlphaFold have allowed
machine and deep learning
researchers to predict the structure
tools that can be used for
of potential drug molecules
tr
molecular datasets
DeepTox
A pipeline for predicting
chemical compounds' potential
toxic effect
Preclinical Screening
RF-Score
A drug's absorption and passage
One of many tools that predicts
through the body is a function of its
binding affinity between
physiochemical properties. These
small molecule drugs and
properties can be predicted using
macromolecular targets
ML methods to create safer and
more effective compounds.
a
4x
100,000
Sumitomo Dainippon Pharma worked with
Insilico Medicine said that their
Exscientia to identify DSP-1181 for obsessive
compound INS018_055 is the first fully AI
compulsive disorder 4 times faster than a typical
generated
drug. It targets a condition called
drug development timeline – taking less than 12
idiopathic pulmonary fibrosis, which affect
months vs an average of 4.5 years.
100,000 people in the US alone
g l
hi
Lab-on-a-chip
Lab-on-a-chip technology combines
laboratory tools onto a small chip
ne
The technology works through microfluidics
The chips are commonly linked to
smartphones or computers where data
analysis using AI can take place.
The scale, throughput and cost-effectivenes
that lab-on-a-chip designs enable can
overcome one of the key challenges facing
AI: generating appropriate data.
The Challenges Facing AI in the Lab of the Future
The Pistoia Alliance’s report also looked at what their life science leaders thought were the greatest barriers to their
adoption of AI and other lab digitization. The graph below shows the percentage of respondents that identified issues
as one of the top three obstacles.
Unstructured data
58%
Unstructured Data
Even if data is available to train and power AI
systems, it still has to be in an appropriate
Data silos/no access data
format for the AI to parse. Messy datasets, like
66%
unclear handwritten notes, compromise the
usefulness of tools like NLP.
FAIRification of data
23%
Data Silos/No Access to Data
Inability to share/transfer analytical methods
Data silos and access problems are common
14%
within bulky company structures. Maximizing
AI’s potential requires different sources
of data to be made available for training
Ontology management
and analysis; cracking open these silos is
essential work.
17%
Lack of metadata standardization
42%
Lack of Metadata Standardization
AI systems are useful because they
Lack of infrastructure and tooling
analyze not just data, but also the data that
28%
provides details about that data – metadata.
By investing in informatics solutions like
laboratory information management systems
Resistance to data sharing/collaboration
(LIMS), metadata can be organized and
48%
mandated into lab practices.
Lack of knowledge and expertize
28%
Resistance to Data Sharing and
Collaboration
Lack of data governance within organizations
Data systems can be upgraded overnight;
30%
changing attitudes towards data sharing
can be a generational task. Academics
and researchers taught to preserve their IP
Other
and afraid of being scooped can block the
8%
availability of data for AI systems.
Sponsored by
References
www.technologynetworks.com/drug-discovery/articles/promises-and-challenges-of-target
1. Pistoia Alliance. Lab of the Future Report 2023.Pistoia Alliance. https://www.pistoiaalliance.org/
based-
drug-discovery-355809. Published 2021. Accessed October 4, 2023.
lab-of-the-future-report-2023/. Published 2023. Accessed October 4, 2023.
10. Lu M, Yin J, Zhu Q, et al. Artificial Intelligence in Pharmaceutical Sciences. Engineering. 2023.
2. Devereson A, Macak M, Nagra N, Idoux E, Stanzl E. AI in biopharma research: A time to focus and
doi:10.1016/j.eng.2023.01.014.
scale. McKinsey & Company. https://www.mckinsey.com/industries/life-sciences/our-insights/
11. Rácz A, Bajusz D, Miranda-Quintana RA, Héberger K. Machine learning models for classificatio
ai-in-biopharma-research-a-time-to-focus-and-scale. Published 2022. Accessed October 3,
tasks related to drug safety. Mol Divers. 2021;25(3):1409-1424. doi:10.1007/s11030-021-
2023.
10239-x.
3. Sultana A, Maseera R, Rahamanulla A, Misiriya A. Emerging of artificial intelligence and
12. Sultana A, Maseera R, Rahamanulla A, Misiriya A. Emerging of artificial intelligence and
technology in pharmaceuticals: review. Future J Pharm Sci. 2023;9(1):65. doi:10.1186/s43094-
technology in pharmaceuticals: review. Future Journal of Pharmaceutical Sciences.
023-00517-w.
2023;9(1):65. doi:10.1186/s43094-023-00517-w.
4. Chassagnon G, Vakalopolou M, Paragios N, Revel MP. Deep learning: definition and perspectives
13. Sumitomo Pharma. Sumitomo Dainippon Pharma and Exscientia Joint Development New Drug
for thoracic imaging. Eur Radiol. 2020;30(4):2021-2030. doi:10.1007/s00330-019-06564-3.
Candidate Created Using Artificial Intelligence (AI) Begins Clinical Study. Sumitomo Pharma.
5. Liddy ED. Natural Language Processing. In: Drake M and Maack MN, eds. Encyclopedia of Library
https://www.sumitomo-pharma.com/news/20200130.html#:~:text=DSP-1181%20was%20
and Information Science, 2nd. Ed. NY. Marcel Decker, Inc. 2001.
created%20through,AI)%20platform%20for%20drug%20discovery. Published 2020. Accessed
6. Khan AI, Al-Habsi S. Machine Learning in Computer Vision. Procedia Comput Sci.
October 4, 2023.
2020;167:1444-1451. doi:10.1016/j.procs.2020.03.355.
14. Insilico Medicine. First Drug Discovered and Designed with Generative AI Enters Phase II
7. Biron L. Meet the Autonomous Lab of the Future. Lawrence Berkeley National Laboratory. https://
Trials, with First Patients Dosed. Cision PR Newswire. https://www.prnewswire.com/apac/news
newscenter.lbl.gov/2023/04/17/meet-the-autonomous-lab-of-the-future/. Published 2023.
releases/
first-drug-discovered-and-designed-with-generative-ai-enters-phase-ii-trials-with
Accessed October 4, 2023.
first-
patients-dosed-301862737.html. Published 2023. Accessed October 3, 2023.
8. Lawrence Berkeley National Laboratory. The Materials Project. Lawrence Berkeley National
15. Kuru CI, Ulucan-Karnak F, Akgöl S. 4 - Lab-on-a-chip sensors: recent trends and future
Laboratory. https://next-gen.materialsproject.org/. Published 2022. Accessed October 4, 2023.
applications. In: Barhoum A, Altintas Z, eds. Fundamentals of Sensor Technology. Sawston, UK.
9. Tay, A. Promises and Challenges of Target-Based Drug Discovery. Technology Networks. https://
Woodhead Publishing; 2023.
Sponsored by
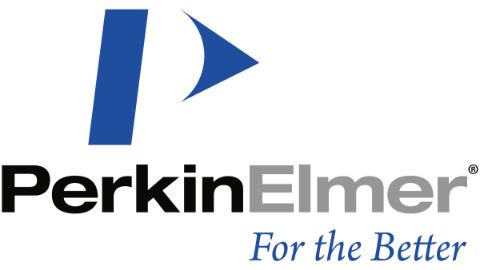
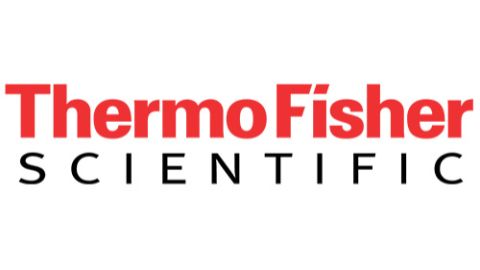
Download the Infographic for FREE Now!
Information you provide will be shared with the sponsors for this content. Technology Networks or its sponsors may contact you to offer you content or products based on your interest in this topic. You may opt-out at any time.