The Spatial Perspective With Professor Emma Lundberg
In the captivating realm of spatial biology, there are few researchers who have made a mark as indelible as Professor Emma Lundberg.
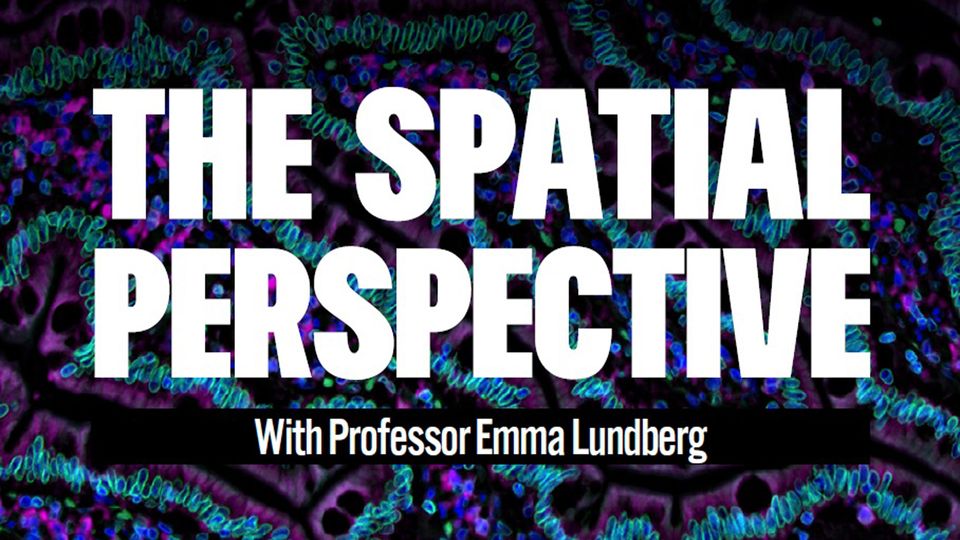
Complete the form below to unlock access to ALL audio articles.
In spatial biology, scientists investigate the organization of cellular components in an effort to understand how their arrangement influences the cell’s function as a whole. The spatial distribution and organization of biomolecules such as DNA, RNA and proteins directs cellular responses. Dynamic alterations in this spatial organization – in response to internal or external stimuli – can subsequently change or disrupt these responses, potentially causing disease.
Scientists have endeavored to peer into cells and disentangle their complex molecular makeup for hundreds of years. But only with the development of technologies such as advanced microscopy, high-throughput sequencing and computational image analysis could the spatial perspective be truly realized. Dr. Emma Lundberg, professor of bioengineering and pathology at Stanford University and the KTH Royal Institute of Technology, and director of the Cell Atlas portion of the Human Protein Atlas, has been a key figure in such developments.
As a postgraduate student at KTH, Lundberg first became interested in the rich data sets produced by bioimaging studies. Over the years that have followed, she has carved a career rich in scientific discovery, pioneering novel techniques for spatiotemporal analysis of proteins, receiving several prestigious awards along the way.
It hasn’t always been an easy feat, however. As you’ll read, Lundberg has faced opposition at times, particularly when trialing novel experimental approaches and strategies to encourage public engagement in science. Thankfully undeterred, she continues to harness cutting-edge technologies – most recently artificial intelligence (AI) – to navigate the spatial realm.
In an interview with Technology Networks, Lundberg discusses the evolution of spatial biology and her career, outlining how spatial insights can provide a deeper appreciation of the complex inner workings of cells.
Molly Campbell (MC): Can you talk to us about your career evolution – what led you to become interested in spatial biology?
Emma Lundberg (EL): I think a lot of what has happened in my career is serendipitous to some extent. I completed a Master of Engineering degree at the KTH Royal Institute of Technology, and during that time I was pretty set on pursuing a career in pharma – I’ve always been very interested in the human body. During my final thesis project, I worked within a research group that was generating small alternative scaffold binders that could be designed to bind to any potential protein, and I had so much fun. That was when I decided to do a PhD, which I hadn’t considered before, and was offered a position to continue the work from that final project.
During my PhD, I spent time doing phage display, generating binders and learning deeply about proteins, which is probably where my interest in proteins – as the versatile and multifunctional molecules that they are – emerged. My PhD offered a solid foundation for what would become my research area of interest, as I did a lot of microscopy work. I was trying to generate binders that could also be used in imaging applications, and I am a visual person with a visual memory, so I enjoy working with images. In microscopy studies, you might have terrible results from an experiment, but that data can still look beautiful – it really appealed to me. I was also fascinated by the richness of the information that you can obtain through imaging studies, and how complex it can be to actually capture that information.
During the final year of my PhD, I started working part-time as a group leader for the Human Protein Atlas (HPA). I had been considering moving abroad to complete a postdoctoral position, but I was offered the group leader position to set up and build the subcellular localization pipeline of the HPA. That’s how it started, and from then on, my focus has been on spatial proteomics.
Professor Emma Lundberg. Credit: Professor Emma Lundberg.
MC: How was that experience – working on the HPA in the early days?
EL: It was super exciting. From a scientific perspective, it was a very unique project at that time, both in the world and in Sweden. It was almost a hybrid project that crossed industry and academia because it was so large scale, and our goals were long-term. For me, it was a very inspiring environment and a unique opportunity. It was difficult to complete a PhD and immediately become a group leader, but it was also a wonderful opportunity.
MC: You recently joined the Stanford bioengineering department. What inspired this decision?
EL: Stanford is a wonderful place, with a lot of inspiring and creative research going on. I had spent many years in the same department at KTH, where I also did my master’s and PhD, so it was time for a change, and I felt very inspired by the environment at Stanford.
MC: How would you describe what spatial biology is?
EL: Everyone knows that humans have specialized organs; we have a liver, we have a brain and we have a heart. These are entities that are specialized in a certain function. The same goes for cells. In cells, there are organelles and increasingly smaller systems with specialized functions. These systems are well partitioned in space – they are physically separated from each other. Through understanding how the cells and the proteins in the cells function, with the spatial context, we can better understand how cells function as a system.
Another way to think about spatial biology is represented in our 2017 Science paper, where we show that half of all human proteins are in two or more compartments at the same time. This has often been neglected, as people tend to want to think that there is one gene, one protein and one function. That’s probably not the case most of the time, and in fact the protein could be in two different places performing two different functions. When we think about it from that perspective, you could explain spatial biology as though you are looking at a house or a village. There are different rooms, different compartments, and if a protein is in the “kitchen”, we might assume it is doing kitchen duties, whereas if it’s in the “laundry room”, it’s doing laundry – and if it’s in between, well perhaps it’s doing both.
MC: In your opinion, what are some of the most interesting applications of spatial biology?
EL: Of course, I would be inclined to say that the work our laboratory is doing is very interesting. We are trying to understand the spatial distribution of all human proteins with the goal of building a spatiotemporal model of all human cells. This work can help us to address the question of how the cell functions as a system. If we have that knowledge, can we build virtual cells?
There are many, many interesting questions in spatial biology. A lot of diseases start with a protein being in the wrong place, or in the wrong place at the wrong time, which can have major functional consequences.
I think, in general, connecting location to function is broadly applicable to many questions in biology that can lead to thousands of interesting applications.
MC: Can you talk about key milestones for spatial biology that have occurred since the field emerged?
EL: First let’s discuss the HPA, where there have been many milestones. Key examples are the ability to produce and validate antibodies at high capacity. Without that, we wouldn’t be where we are today. For the Subcellular Section of the Human Protein Atlas, which I am leading, a very important milestone occurred back in the day when we had just started the project and were unsure about what microscopes to use. This might sound trivial today, but it was a key decision to make in 2007. The pharmaceutical industry was using high-content screening microscopes, but they would just image everything with the exact same setting – we were moving one protein at a time, blindly, and there is so much variability in protein expression. I figured that this wouldn’t work for the project.
I decided to take regular confocal microscopes and position them upright – essentially turning them the other way around to their regular configuration – and automate them ourselves. This was met with a lot of skepticism at the time. I was a new group leader and I was nervous as to whether this was the right decision or not. In the end, it was a great decision. We used the same microscopes for 15 years afterwards. Another milestone was being able to automate the imaging and the sample preparation steps so that we could prepare 500 samples a day and image them. We could do this research at a scale that had never been achieved before and with quality control measures in place.
I think the next milestone would be the series of Science papers that we published from the HPA. There are different groups within the HPA, and we work in small teams in close connection with each other. It’s almost a “mini industry”, if you will.
First, we published Tissue-based map of the human proteome in 2015, which was the first paper that outlined where proteins are expressed in human cells. Then we published A subcellular map of the human proteome in 2017. This was a series of milestones achieved in short succession, but keep in mind that we had been working on this research for over a decade.
Since then, we have achieved several further milestones, particularly in relation to the way that we operate. We have transitioned from a brute force approach of “let’s just map everything” to addressing more targeted research questions. An example research study is our work published in Nature back in 2021, where we explored protein and RNA expression in relation to cell cycle progression. This required more advanced assays, we included single-cell transcriptomics and the work was more tailored. It was also conducted at high capacity.
A more recent milestone that I believe will change a lot of aspects of spatial biology going forward is our ability to use machine learning to harness the information we obtain from the images. Instead of using our eyes to look at an image and say, “Oh how beautiful, this image is located in the mitochondria”, we can use AI to embed that information. We can turn an entire image into a vector of numbers that store information about that image and model the cell. Then we can start to integrate that data with other molecular measurements. For example, we built a structure of functional systems in a cell, demonstrating that we can model from the images. There is a lot of cool work going on too that we haven’t published yet.
Generally, spatial omics might seem like a new field, but people have been looking through microscopes for a long time – so it is actually quite old. We have been visualizing proteins and molecules for years, but our capacity to do this at a large scale has dramatically changed in the past two to three years. We are at an inflection point, particularly in spatial transcriptomics where technologies have really advanced. Now, we can visualize RNA in situ. You can look at hundreds and thousands of RNAs and explore gene expression patterns with high sensitivity and high resolution across cells and tissues. This ability has really changed the way that we can do biology; we can look at tumor heterogeneity, for example, and understand treatment responses based on differences in gene expression changes across regions of the tumor.
An immunofluorescence image of cells derived from osteosarcoma. The DNA in the cell nucleus is shown in blue, and cytoskeletal elements called microtubules in red and actin filaments in green. Credit: The Human Protein Atlas.
Spatial proteomics is more technically complex because we can’t amplify proteins, so we’re a couple of years behind – but we’re getting there. There are many interesting technologies for multiplexed imaging that are established and emerging, and we’re seeing more people adopt them. So, we’re perhaps experiencing an inflection period as well. I’m very interested to see what happens with protein sequencing technologies and their impact on the field.
MC: Much of science requires us to use our imagination to put information into context. It must be appealing in spatial biology to see your results in right there, in front of you.
EL: Exactly. There is a microscopy conference called Seeing is Believing, and I think to some extent that is true – it is easier to believe something if you see it. However, it’s also important to not be fooled by the fact that you see something. It can be a double-edged sword.
MC: You’ve spoken about the positive impacts that machine learning can have on spatial biology. Do you see any adverse effects at this stage?
EL: Absolutely. We have a model that can generate synthetic microscope images that you really can’t tell apart from real images. You can see how such a model might have great usage as a foundation for whole-cell modeling, to predict where proteins that you haven’t stained are, for virtual microscope experiments, etc. But of course, you can also see the potential for misuse of such models. I think this issue extends beyond microscopy. I am currently working with a group in bioethics to think deeper about what the problems are and how we can address the issue of misuse.
MC: Can you talk about some of the key challenges that you face, perhaps in your own field but also speaking broadly about the spatial biology field?
EL: We can’t amplify proteins which is a huge challenge and one that is really hard to overcome. I think another core challenge when studying proteins compared to transcripts is the dynamic range of proteins. Some proteins are very abundant, and some proteins exist as a few copies that are regulated with high fidelity and are incredibly important for cell function. That is a difficulty we face with the technologies of today – maybe we still cannot detect low abundant proteins, or we only detect the high abundant proteins.
In my everyday work, study design can be a challenge. Historically – and still today – we are working with antibodies to target proteins, which means we need to know what we want to target and study; we need to have a hypothesis. Compared to mass spectrometry, where you can obtain an unbiased total protein readout, this can be difficult. We want to be unbiased, but we still have to make a targeted selection. To circumvent that problem, we have been working with a technology that I am really excited about, which combines antibody-based methods and mass spectrometry, called Deep Visual Proteomics. We do highly multiplex imaging first to visualize all the cell types, then we use a laser microdissection microscope to cut out the different cell types before we do ultra-high mass spectrometry. The end result is the ability to project deep, unbiased measurements onto the original image. It’s not straightforward, but it’s super fun. What’s nice is that we’re using the imaging to guide the sample selection and then we get unbiased readouts.
MC: You are clearly passionate about engaging the wider community with your work. Can you tell our readers about the citizen science project, “Project Discovery”?
EL: We have generated millions of images in the HPA, and previously we annotated them all manually. This is fun but very time consuming.
We reached a point where we realized that there is more information than what we can annotate in these images. We were thinking: How can we get the general public interested in this project to help us? I have a genuine belief that a lot of good things come from interactions between scientists and the general public – they are fun, but they are also inspiring.
We were encouraged by previous projects that have asked the general public to support research efforts through playing a game. We teamed up with game developers at a company called MMOS and agreed that we would try to find an existing game and inject our images into that game.
We ended up working with CCP Games in Iceland, so it was a three-part collaboration between CCP games, MMOS and us. For about a year we would meet every week and build the project, it was very fun – super different to other research projects! We built a “mini-game” called Project Discovery within an existing game called EVE online. While players are waiting for their friends in the game’s waiting room, they could play Project Discovery, and were made aware that they were contributing to science. I was also part of the game and had an avatar created called Professor Lundberg – she even has freckles. It was quite freaky!
Initially, the scientific community was divided in their opinions about the game – some people thought it was cool and fun, others were questioning why we were doing it. We were nervous when we launched, but it was a great success. We reached our yearly goal within a week. It was obvious to us that this was a great approach to citizen science and to encourage the public to participate in scientific research.
With projects like this, you can't just develop it and leave it there – you have to engage with it, you have to answer questions in forums and participate, so it is a lot of work. My students were in the game while they were at the lab. The gamers did very well, and we could use the data to update the Protein Atlas. A lot of people reached out to us to let us know that they gained a passion for education and STEM because of the game, it was striking and really quite heartwarming. We hadn’t designed the game with that in mind, but it struck me that you can fulfill many purposes through an initiative like Project Discovery.
MC: Were you happy with the avatar?
EL: I was, but we had to change the outfit.
MC: Are there plans for any similar projects right now?
EL: Not at the moment, but it’s one of the reasons that I think it is really fun to be in Silicon Valley right now. It is a great place to be – I definitely have some ideas cooking.
MC: What do you envision the future of your field will look like?
EL: Short term, I think we are seeing a very rapid and focused development towards single-cell proteomics, which I believe will change the way we do proteomics and lead to a lot of insights. I think it will create a fundamental shift in the way that we work.
When I talk about proteins, basically everything that we are doing is simplifying biology to consider one protein per gene, but we know that different proteoforms exist due to mechanisms such as post-translational modifications. We have a long way to go in terms of understanding how proteoforms modify cell function. This is a long-term challenge that I think novel technologies, such as protein sequencing and advanced mass spectrometry, can help with.
I think we’re at the brink of a new era when it comes to structural cell modeling. If you think about the cell as having lots of building blocks, we know the genome project showed us the parts list, and then through these various atlas projects we have made an inventory of the parts list. What we don’t have is enough information on the assembly of the parts and how it leads to this functional cell machine. I hope that within 10 years we’ll have a model of the assembly of a cell. I envision a future where we can build virtual models of cells and use them to simulate how you would respond to a drug before being exposed to that drug. Even though we’re far from that point, I think we’re going to see how AI will propel this field forward in the coming years.
Professor Emma Lundberg was speaking to Molly Campbell, Senior Science Writer for Technology Networks.