What Are Ontologies and How Are They Creating a FAIRer Future for the Life Sciences?
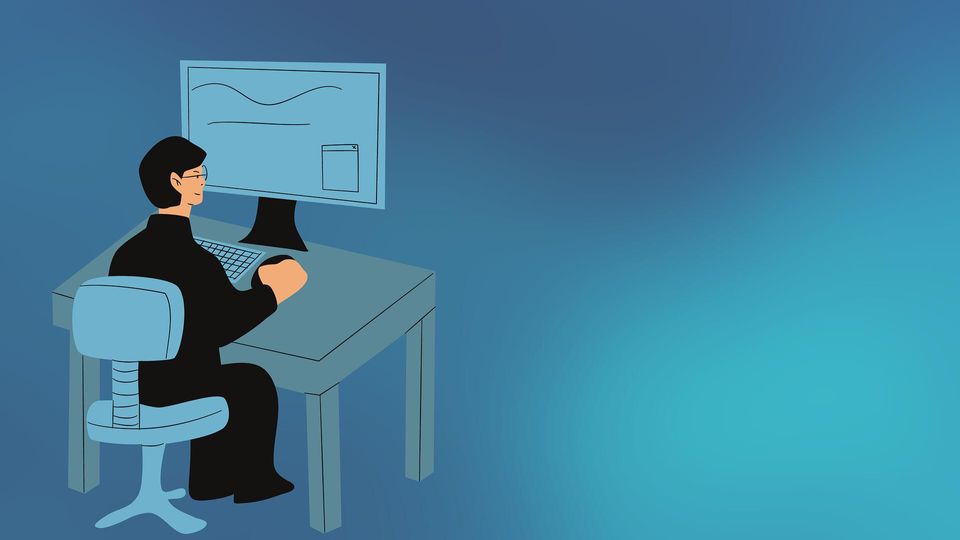
Complete the form below to unlock access to ALL audio articles.
In recent years, drug discovery has charted a new course towards targeted precision therapies as we enter the age of the niche-buster drug – i.e., drugs targeted at under-served disease subpopulations – and personalized medicine. Initiatives like the 100,000 Genomes Project and Precision Medicine Initiative offer an exciting glimpse into how targeted approaches can advance patient outcomes, as well as improve our overall understanding of human biology. At the same time, these projects underline that the success of precision medicine will rest on companies being able to harness vast volumes and variety of data – including published literature, proprietary and experimental data, as well as patient and healthcare records.
Artificial intelligence (AI) offers life science companies an attractive option for extracting knowledge from complex and varied data, and many are exploring how the technology can accelerate their research initiatives. But there’s an important caveat – much of the data available today simply aren’t AI-ready. Data are siloed and stored in myriad formats with insufficient metadata, making it difficult to retrieve, analyze and share. This means many are setting themselves up for failure if the data feeding the AI model aren’t high-quality, trusted and machine-readable. A key prerequisite for AI, therefore, is to make data FAIR (Findable, Accessible, Interoperable, Reusable). This is where ontologies come into the picture.
What is an ontology?
Ontologies are human-generated, machine-readable descriptions of knowledge, and can be a critical tool in the big data challenge of making data FAIR. However, outside of expert circles, there is little understanding of the scientific and commercial value ontologies can bring, or even awareness of their existence at all, which can hinder the success of data projects.
Broadly speaking, ontologies describe “types” of thing (also known as classes) and the relationships between them. For example, an “egg” is a type of “food”. We might then have subtypes according to how the egg is prepared – e.g., fried, scrambled, poached. The classes may have textual definitions that a human can use to understand what the class contains, as well as synonyms and relationships to other classes. For example “hen egg” derives from “hen”. Synonyms can be useful in understanding the different ways the things represented by an ontology class can be represented. In the life sciences, one example is different ways to refer to a gene, such as PSEN1, which can also be PSNL1 or Presenilin-1.
Ontologies strive to be a community consensus view of a domain, which is continually evolving and is updated with our latest understanding of the world. Many ontologies that exist in the biomedical domain are publicly available and maintained by the community, for example the Human Phenotype Ontology (HPO) or the Gene Ontology (GO). So, if a new synonym of PSEN1 is identified, experts in that domain – geneticists – update the ontology to incorporate it. In the context of life sciences, domain expertise is essential as human biology is far more complex than our egg analogy. Biomedical ontologies will be powering algorithms across drug discovery and delivery that will be making important decisions about diagnoses or which drugs a patient should receive, so it’s critical they are accurate.
How do ontologies FAIRify to overcome big data challenges?
Life science companies currently face a two-pronged challenge: FAIRifying their legacy data and ensuring new data generated are also FAIR. Curating data with domain-specific ontologies helps to overcome these challenges by structuring data in a way that ticks the FAIR boxes.
Unstructured legacy data pose both an ongoing cost to organizations and a missed opportunity. Much time is wasted searching for and cleaning data for reuse. This productivity loss in turn slows time to market and ROI. Additionally, potentially valuable scientific insights remain obscured when information is not annotated and organized. Where metadata is available it may not always be consistent – there is often a lack of standards or common terminologies applied across an organization. This prevents data being easily discovered, integrated and reused by scientists.
Compounding the challenge of legacy data, newly generated data is often not being captured in a FAIR-compliant manner either. This can lead to up to 85% of all research simply being wasted due to a lack of data standards. Ensuring that data is “FAIR from birth” is critical in preventing them becoming part of the vast amount of legacy data companies are already contending with. For example, data entered into electronic notebooks (ELNs) are typically free text, making these datasets very hard to search in the future. One solution to this could be smart data entry, where scientists use for example ontology-powered type ahead when inputting assay information, such that data are normalized with ontologies at the point of entry.
Ontologies provide unique identifiers with associated names and synonyms which can help with the normalization of scientific language – sometimes referred to as “things not strings”. Tagging data with these identifiers makes it easier to search and analyze for scientists, as it includes results that contain synonyms or associated terms that the ontology recognizes as being related to the search query. Furthermore, since ontologies are based on an accepted community model, data are presented in a way that is widely understood, reducing the number of instances competing terminology is used.
Crucially, ontologies ensure data are machine-readable, harmonizing them for analysis with AI and machine learning. With data structured in an ontology, companies can be sure their algorithms are learning from the full picture of information, reducing the risk of error, and improving accuracy of results.
Case study: an ancient use case makes data relevant for today
With the right expertise, ontologies can be applied to any legacy data. A recent project tagged texts relating to traditional Chinese medicine (TCM) to open up new resources for modern biomedical scientists to utilize. TCM is of growing interest, with its domestic value expected to rise to US$107 billion by 2025. The field has ancient texts with numerous spellings, synonyms, translations and symbols, and multiple ways to refer to the same medicine.
The researchers used their domain expertise to build an ontology that links traditional Chinese and modern English language names for compounds to empower researchers with knowledge about the ingredients of specific TCM compounds. The ontology has made hundreds of ancient resources FAIR and is being used to power algorithms that will enable the development of innovative new medicines using knowledge from ancient medicines. This is just one example that demonstrates ontologies are not just a way to improve productivity, but have real implications for medicine development.
To deploy AI, it’s time to deploy ontologies
If your organization wants to use AI to drive precision therapy breakthroughs, it’s time to start getting your data in order. Doing so not only will accelerate R&D, but will also drive business value – The European Union estimates that not having FAIR data costs more than €10.2bn every year. FAIR implementation unlocks the long-term potential of data, enabling faster and more detailed analysis. For the business, there are serious productivity gains to be had. And for the most important stakeholder of all – the patient – there are new paths identified in the quest to create new targeted therapies and better outcomes.
Ontologies will be at the center of this transformation, de-siloing, standardizing and harmonizing data sources to transform unstable text and images into data that powers discovery. To learn how to kickstart your ontologies project, watch this space for the second article in this series.